11 minute read
A pool of valuable data
Next Article
decision to take action and that action fails, or likewise, if failure results from deciding to take no action, against the recommendation of the digital twin. Costs are also an issue, particularly in an industry that tends to remain comfortable with reactive maintenance models and condition monitoring, and there are no generally accepted, accurate ways of calculating what kind of savings such systems could offer, if it could alter and inform the ‘way we have always done things.’ Indeed, it has taken much learning to make these digital tools, and it will doubtless take much learning before we can use them effectively in driving efficiencies into our practices as well.
Currently, digital twin technologies find their use cases across a wide variety of business and industry applications 1 : > Manufacturing: Substantial influence on maintenance and product design. Industrial IoT: Monitoring, tracking, and controlling of systems. Healthcare: Reducing expense and providing tailored support to patients. Smart cities: Planning, building, administration of resources, and decreasing environmental impact. Automotive: Performance efficiencies, behavioural and functional modelling. Retail: Customer experience enhancement, inventory control, and modelling of consumer behaviour.
Indeed, just based on the above list, we can, and should, imagine a tremendous number of use cases for digital twinning in offshore wind, both for fixed and floating (FLOW) facilities. Engaging digital twinning is important to offshore wind because of one major aspect that makes offshore wind unique compared to that list: offshore wind operations occur in remote, harsh, risk-laden marine environments. Anything that reduces risk in this type of environment by cutting the need for frequent transit is worth exploring, especially considering the long-term commitments that have been made in offshore wind – normally 25 years for a fixed-foundation farm in the North Sea.
Figure 1. Knowledge management sophistication.
By combining known offshore wind industry pain points with conceptual use cases for digital twins, we can begin to see a number of solutions forming on the horizon, some of which are achievable today with modest cost and effort. Also, if we accept that there are only two fundamental ways of creating more value in offshore wind – making more electricity or spending less money – and it is accepted that the maximum generation capacity is capped by the physical limitations of the farm itself and the conditions in which it operates, cost-savings and efficiencies as primary drivers for the industry should be turned to, specifically for maintaining asset availability. With regard to addressing pain points, this article will briefly examine digital twinning for use in data aggregation, condition monitoring, and driving efficiencies into maintenance regimes using optimisation techniques, as they relate to cost-savings. It is worth mentioning that the planned zero-subsidy transition over the next four years also helps add a sense of urgency to the mix.
Data aggregation and knowledge management
In interacting with numerous entities across the offshore wind space on a range of different levels and topics, the one overriding pain point that is repeated in many conversations is ‘just getting all of our information together in one place’ – i.e. data aggregation, then data management going forward once all that data has been aggregated and collated. In offshore wind, this pain point is most acute between phase handovers (i.e. design to construction, construction to commissioning, commissioning to O&M, etc.) and between entity handovers (i.e. designer to constructor to commissioner, between owners, managers, and contractors).
The commonality here is simply that any time data or information gets transferred, some portion of it gets lost in the process. In the field of information systems, prevention of this phenomenon is known as knowledge management (KM), because it deals not just with the transfer of raw data, e.g. from sensors, but also all sorts of other documentation such as design drawings, engineering specifications, inspection reports, commissioning certificates, photogrammetry, survey data, etc. The list goes on if the goal is to create a true digital twin for an offshore asset. KM also goes beyond document control, which helps form the structures and substructures of KM by applying consistent nomenclatures, organising premises, version and permissions controls, and so forth. Likewise, KM must also go beyond simple condition monitoring and reactive alarming in terms of a fourth-dimensional element that tracks condition trends over time, eventually feeding predictive
analyses. Indeed, the relationships in the real world that the twin is to represent in the digital world must be understood, and that understanding must be accurate enough so that the twin is consistently useful to us in the role it is intended to be assigned. Ensuring a twin’s usefulness is the product of sophisticated knowledge management that resides well up the data maturity line, which corresponds to the value that can be generated from the data (Figure 1).
Thus, as is evident in Figure 1, KM has width, breadth, and depth, based on ideas such as data governance, variety of inputs, and contextualisation of data held. However, it also has a fourth-dimension component, because an organisation’s knowledge must be maintained over time to accommodate change and prevent information loss as the level of sophistication in data use is increased.
Once incoming noise has been aggregated and organised in a sensible fashion, that noise has been turned into data. Once the data is organised, that is the creation of information – then analyses can be used to turn information into knowledge. Information is something that value can be created from because actions can often be taken on it – actionable intelligence derived from reactive condition monitoring is a good example – but it is still not enough for a robust digital twin. That fourth dimension is needed from the X-axis to vault us up the Y-axis of sophistication in order to have the ability to engage in hypothetical experimentation, e.g. what adjustments are optimal if I have known degraded fault protection in a number of locations under maxproduction conditions? This upward vault moves us into the area of knowledge transitioning into wisdom, which comes from knowledge organised with experience over time – or in the case of digital twins, machine learning over time to create robust statistical models that can drive the increasingly accurate analyses of hypothetical situations.
Optimisation and cost-savings through digital twins
Returning to the initial list of use cases for digital twins in other industries, common threads and their corollaries within offshore wind can be easily found, expressed in abstract terms that extol benefits such as efficiencies, cost-savings, planning, control, etc. However, it is intuitively understood that the majority of the lifecycle of the asset is spent in the O&M phase, being monitored and maintained. These terms must then apply to O&M as well as the preceding phases, in order to realise long-term value creation through savings. Fortunately, all of these threads apply very well to the types of maintenance processes performed in offshore wind, both routine and nonroutine, and for both planning and execution.
For routine O&M planning and execution across the asset’s lifecycle, opportunities are evident for digital twin application in much the same light as offshore oil and gas. Twins reduce the number of offshore trips required to plan and execute maintenance campaigns of all sizes and durations, because much of the planning can be undertaken onshore, which in turn helps optimise the number of trips required to execute. Additionally, by folding in data science techniques, there can be better prioritisation of the work-packs in the plan. This is based on chosen parameters, such as rate of increase in severity of an anomaly, knock-on effects of degradation or failure in a particular area of the asset, or changes to sea states across the farm based on forecasted weather conditions. For all of these examples, a good digital twin would provide, first and foremost, the reduction of risk to personnel and vessel hire expenses by reducing the overall number of trips offshore. Secondly, it would provide the reduction of the risk of downtime by better understanding the consequences of various failure modalities from the modelling of hypothetical scenarios in each phase of life of the asset.
For non-routine maintenance and emergency response throughout the lifecycle, a viable digital twin can help companies optimise response plans by allowing them to run any number of hypothetical scenarios in advance, so that James Fisher AIS can model how long it will take, from first alarm to resolution, by modelling the effectiveness of different response plans. Likewise, as the model learns over time through the use of machine learning algorithms, it will be able to aid in statistically pinpointing the location of faults based on changes in sensor input contrasted to the historical baseline ‘behavioural patterns’ of the wind farm collected over time and under various conditions. This type of learning could reduce expensive fault locating activities from potentially days to minutes as the accuracy increases with time.
Finally, by integrating packages like SAP and Maximo, the digital twin for the physical offshore asset could easily be tied into a fiscal twin, based on known cost/time/resource input for personnel, plant/equipment, transport, and consumables, as well as to production figures from SCADA and strike price quotes from the market. Applying all of these sources would greatly enhance the cost-savings and provide an additional dimension of decision support during planning and budget allocation projection activities, not just in the O&M phase, but across the lifecycle of the asset.
Case study
Currently, James Fisher AIS has numerous projects running in offshore wind, with one client in particular signing onto a pilot project funded by ORE Catapult to create a digital twin of a wind turbine (Vattenfall), to be followed by a second client’s desire to photogrammetrically capture an offshore substation linked to different assets in the North Sea (SSE, Greater Gabbard), both as proofs of concept. Both projects will then have relevant data and information embedded into the spherical photos using the R2S system, then linked into SAP and/or Maximo for planning and logistical consideration. Likewise, selected portions of SCADA can be integrated into the visualisations in order to provide a more comprehensive creation of value from data, both real-time and historicallyanalytically. The R2S system has been used in oil and gas for just over a decade, and is currently maintaining years of asset data across hundreds of offshore assets throughout the world.
References
1. AUGUSTINE, P., ‘The industry use cases for the Digital Twin idea’, Advances in
Computers , Vol. 117, No. 1 (2020), pp. 79-105.
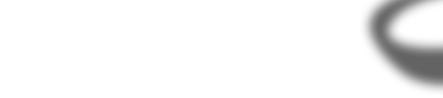

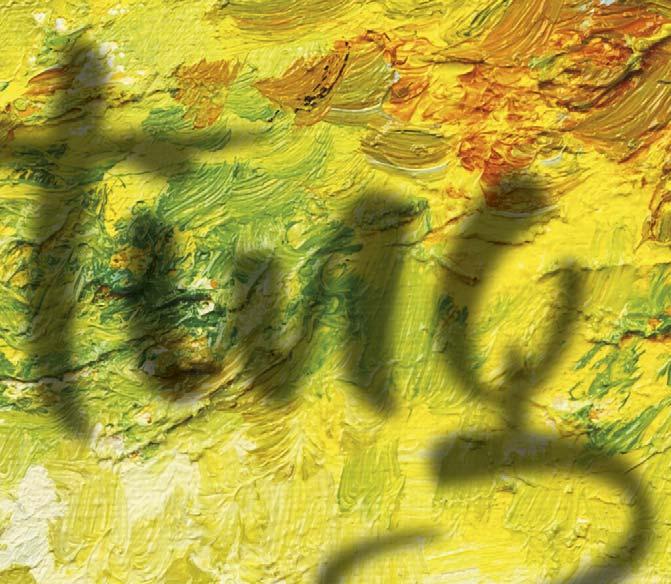
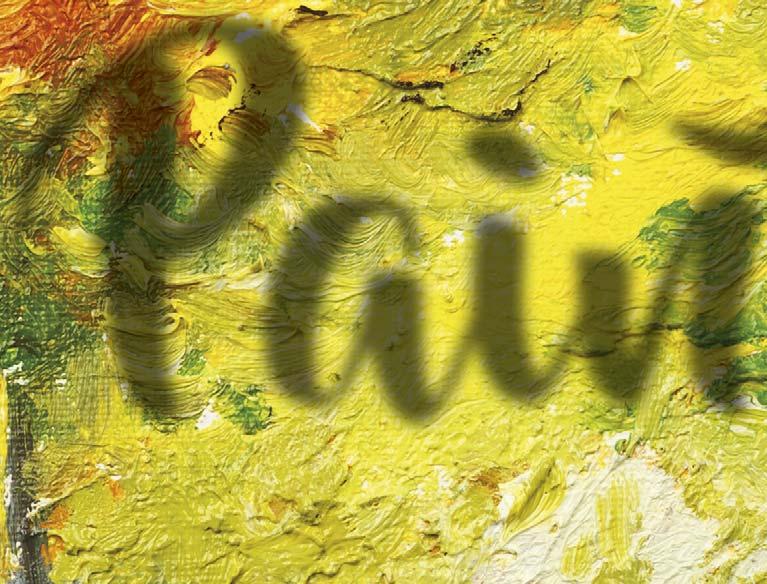
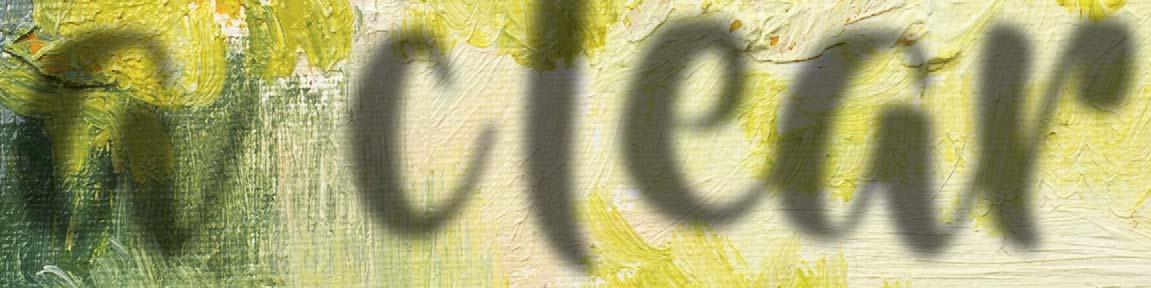
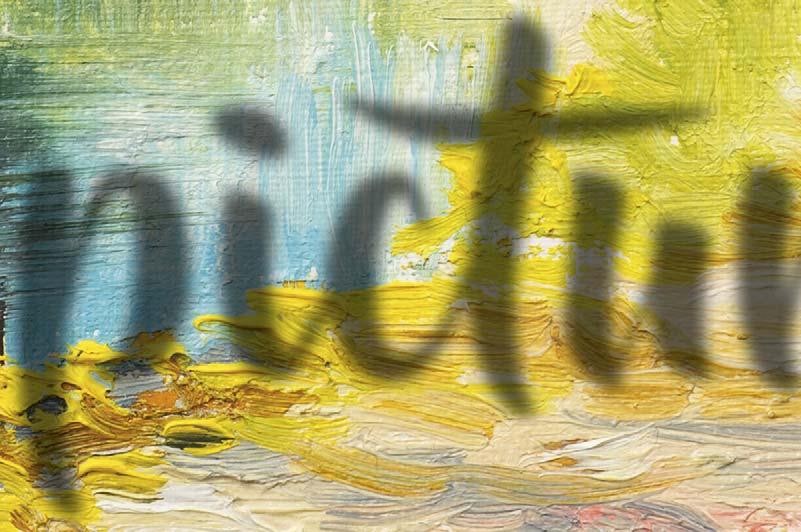
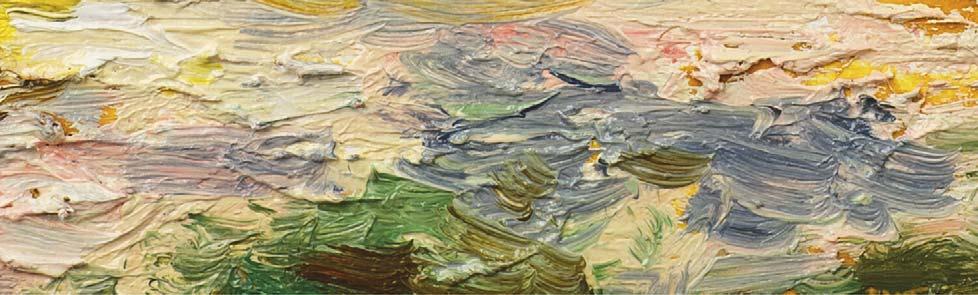
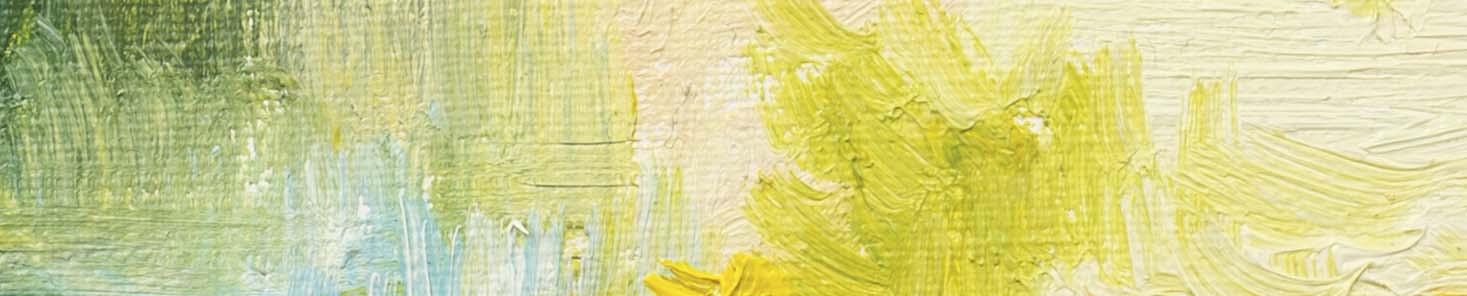
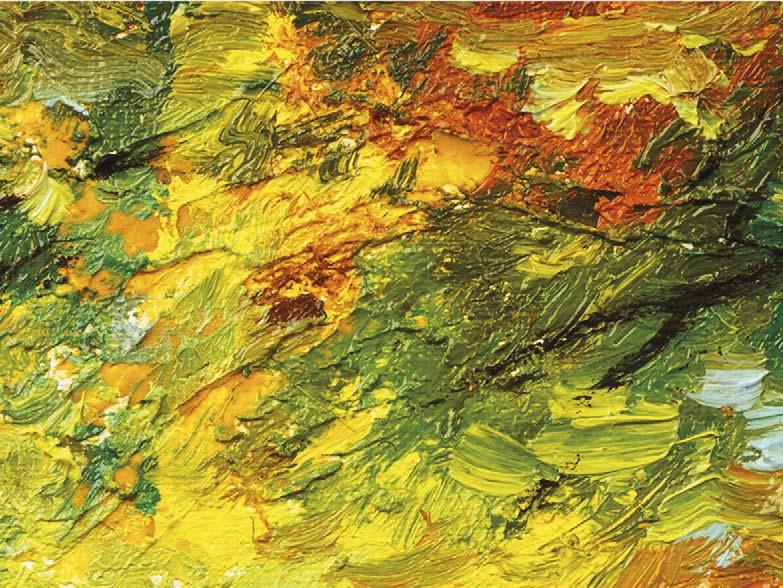

Gareth Brown, CEO, Clir Renewables, Canada, explains how contextualised data can build a true picture of wind turbine performance.
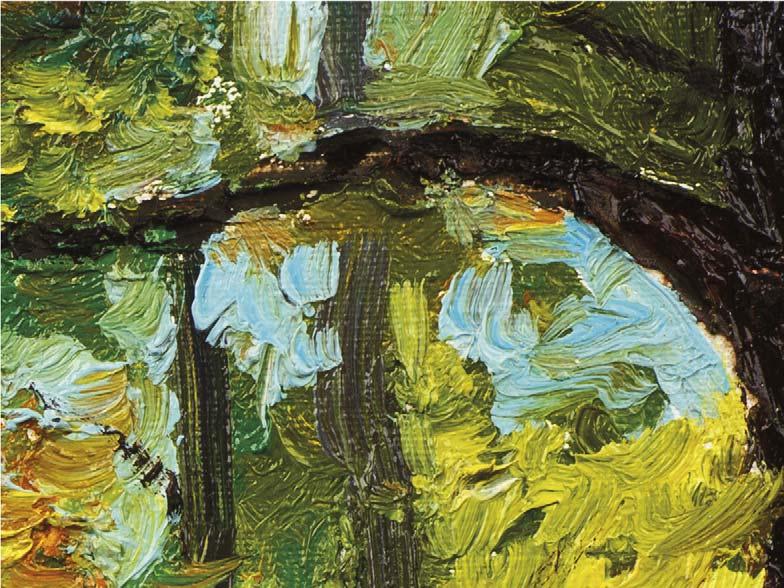
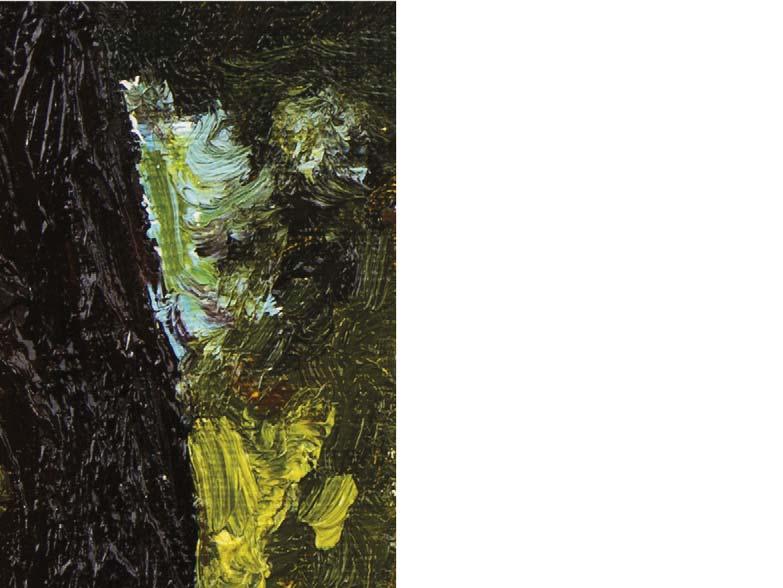
Renewable energy has grown significantly over the past few years, and while COVID-19 may lead to a drop in new installations this year, the industry will continue to see record investment over the next decade. The global economic downturn and coinciding oil price war have driven investor interest in more stable assets, which, in conjunction with continued calls from the public and shareholders for greater corporate commitment to sustainability, have led many institutional investors to back renewable energy.
However, in order for investment to continue to grow, the renewable energy industry must tackle the assumptions holding wind asset owners back from addressing underperformance. A proportion of turbine output does depend on an uncontrollable natural resource. However, the assumption that a low wind resource makes up the majority of underperformance means that hard-to-find faults or failures with turbine technology are often missed, leading to owners losing out on revenue.
Often, by the time that patterns of underperformance are recognised as a technical problem rather than a natural variation of the weather, owners have already lost significant revenue from a fault that could have been easily fixed if identified early.
This is not just an issue for a few unlucky asset owners. At present, the majority are missing out on turbine performance because they do not have a clear picture of the true causes of asset underperformance. The industry must set data in its environmental context in order to understand the true causes of turbine underperformance and rectify them.
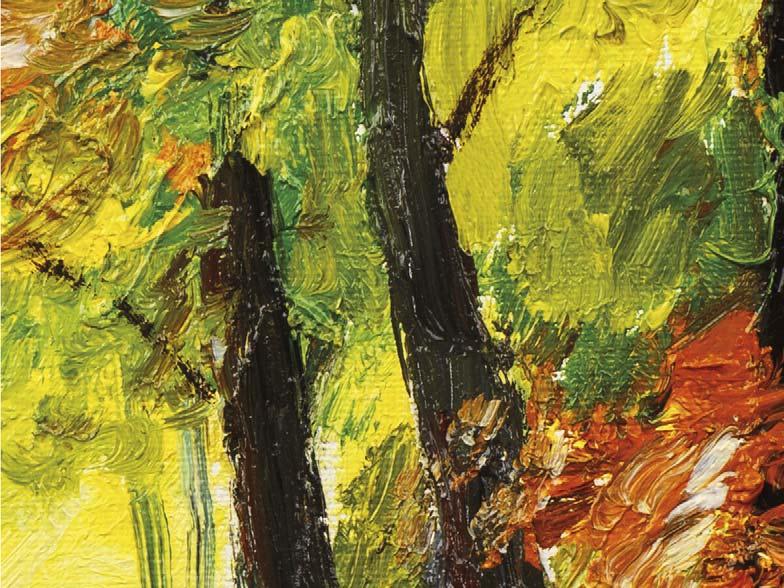
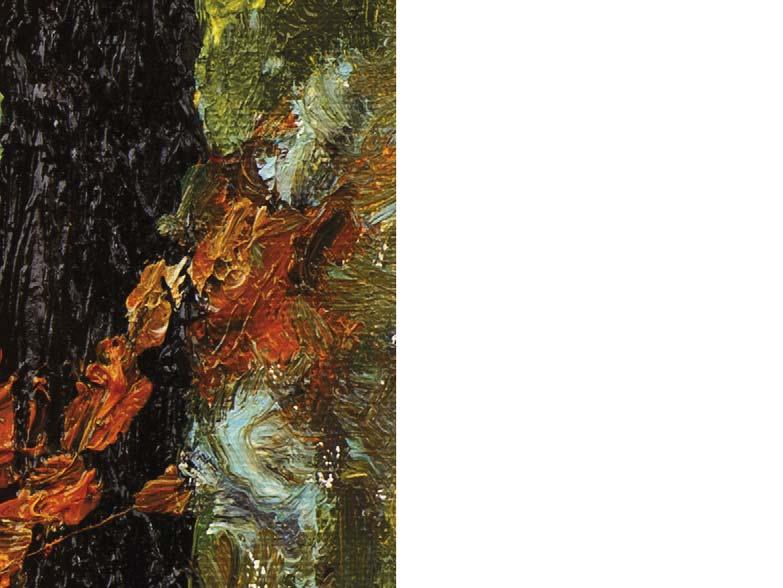
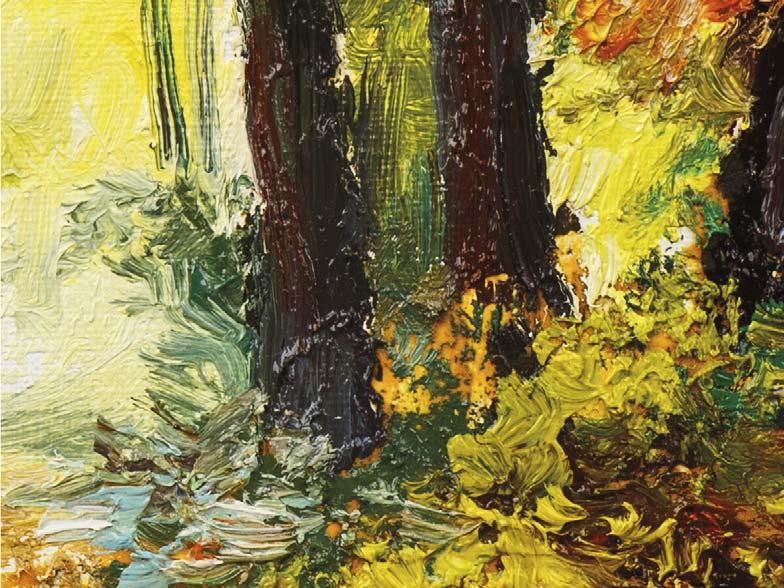
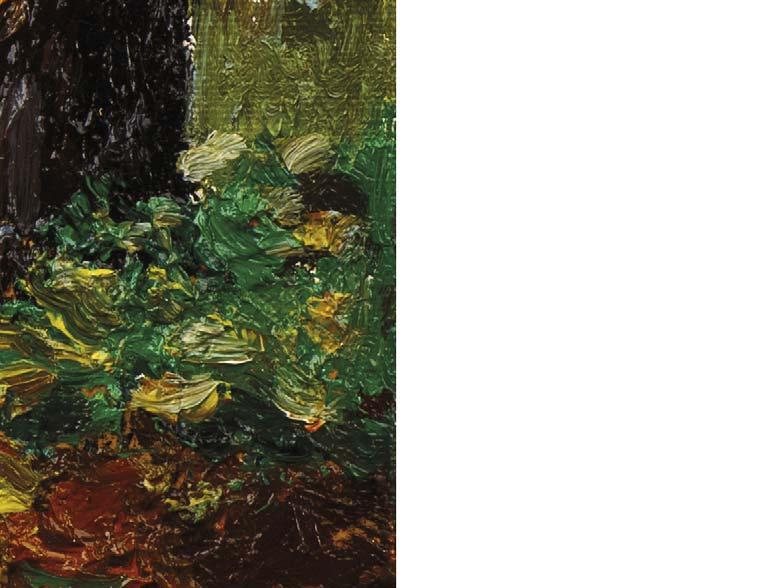
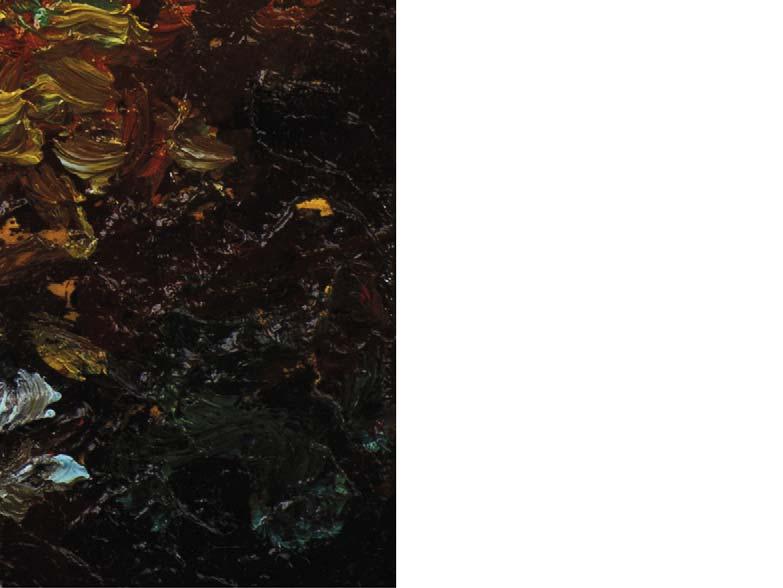
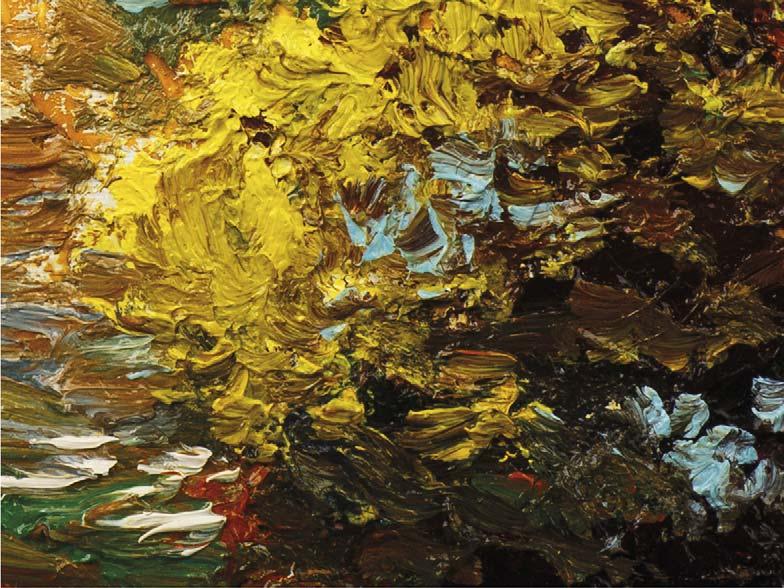
Putting asset data into context
The renewable energy industry collects sufficient data from its projects to identify whether low output is due to natural variations in resource or due to technical faults. However, these data streams are often disparate and disorganised.
The wind industry is unique in the sense that in comparison to conventional power plants, the owner cannot accurately measure resource via a controlled intake. In the context of a wind farm, not only is the resource challenging to measure, it is also impacted by geospatial conditions, whether that is forestry, steep slopes,