Heartcore Capital
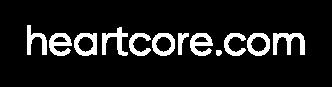
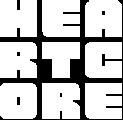
Heartcore Capital
Why AI-enabled software is a catalyst for a new age of human productivity
May 2023
AI will reshape every software category and accelerate human productivity. The advancements in machine learning provide unprecedented ways to augment humans across all professions. Reimagining work will be one of the biggest challenges and opportunities in the coming decades.
AI is undeniably one of the most pivotal technology shifts in recent history. The advent of large models has democratized AI capabilities, making them readily accessible for adoption by all technology companies. Unlike the transition from on-prem to cloud, current incumbents aren't disadvantaged to quickly adopt this new tech. In a game that rewards scale, nimble startups might not necessarily have the upper hand. Nonetheless, there are countless exciting opportunities to build application layer productivity solutions and propel humanity forward with AI-powered software.
In many Western and Asian countries, the looming demographic shifts present a tidal wave that's likely to accelerate the adoption of AI. As companies grapple with challenges to grow their workforce, they'll have no choice but to embrace software automation.
When it comes to AI, it feels like a lot has already been said in recent months, but sometimes a fresh perspective uncovers novel ideas. This report is primarily tailored to founders who want to navigate this technology shift, effectively integrate AI into their roadmap, and steer through the emerging competitive realities arising from deep learning and large models.
Productivity Redefined : AI’s Path To Value Creation
Innovation Landscape: Category Winners & Emerging Innovators
Unveiling AI: Why The Hype & Why Now? 1 2 3 4
Shaping the Future: Research Breakthroughs & Expert Contributions
The "iPhone moment" for AI: ChatGPT reached 1 million users within 5 days
Time from launch to 1M Users for selected technology applications
AI is one of the most significant technology shifts we’ve seen in the last century.
UN population development projections for selected countries (age 20 - 60, Indexed)
Maintaining real GDP growth requires productivity gains per citizen in the workforce
GDP growth will no longer come from an increase in population - AI will have to fill the productivity gap.
Advancements in hardware are converging with AI architecture innovation. This is applied to lots of data.
Architecture (Parameters)
Compute (FLOPs)
Data (Tokens)
Large Models: achieving good outputs for a wide set of complex tasks, despite training on unlabelled data
Large AI models are a major breakthrough for software development.
Better GPUs paved the way for AI innovation, such as LLMs. Once again, hardware advancements were the enabler of software innovation and value creation. This is not dissimilar to technology shifts of the past like on-prem to cloud, and Web to Mobile.
Transformer models are efficient for training on large data sets without human supervision
Transformer-based models have shown to be very efficient in training on GPUs by parallelizing the ingestion of large amounts of data. Attention mechanisms allow the model to focus on specific parts of the input sequence while processing it, thereby improving its ability to understand and generate complex patterns.
"Looking at previous words only”
Luke, I am your
best worst mother
"Looking at all words at once"
Luke, I am your
father
Models like GPT-3 have been trained on terabytes of public text data. These data sets pale in comparison to other text-based content that’s been created by humans. Future SOTA models will be trained on so far untapped non-public and unstructured data.
State of the art LLMs were only trained on a tiny fraction of human created text
Non-public Text data Emails/
Large models are trained on large data sets. But they’re only scratching the surface.
We've seen an arms race to train larger and larger models with more parameters (neurons). Output quality and parameter amount seemed to scale in tandem until 2021. Nowadays, data architecture and training sequences are back in focus in an effort to address surging training costs.
Source: Adapted from StateofAI Report 2022, Nathan Benaiche
Model size is not all that matters.
Companies raced to train their models with more parameters.
We expect impactful LLM innovation to centre around autonomous agents. This will likely allow for another big step in productivity gains.
“Embedding a lot of text”
“Teaching LLMs to perform more than auto-complete”
“Guiding LLMs to give better answers”
“Helping LLMs to operate beyond their training set”
Generative AI is leading towards hyper-personalization, allowing for new business models and value creation, but at the expense of traceability and governance. Increasingly content (from image, audio, and text to graphical interfaces) will only exist for a single human and a single moment.
Read-only Web
1 website for millions of visitors
Web 2.0
Curated content for one user or demographic
Generative Web Ephemeral content for one user
News sites
Corporate websites
eCommerce
Recommendation engines
Social-media feeds
Programmatic ads
Momentary media
Generative UI
Personal Chatbots
Open-ended video games
Large models are commoditizing AI capabilities. Companies providing productivity solutions are now able to adopt a combination of proprietary and thirdparty AI solutions. To achieve industry specific value creation a proprietary approach to finetuning derivative models (L2) and orchestrating tooling is required.
Application layer
Orchestration
L2 – Finetuned models
Operating System
Software
L1 – Large models
Hardware layer
The industrialization of AI-capabilities is an exciting opportunity to create value at the application layer. Despite the justified hype around AI, entrepreneurs will have to be mindful that LLMs are redefining the nature of competitive moats.
Ø User interface
Many successful AI companies follow the same strategy - just from different ends of the stack. LLM providers are building vertical and horizontal application layer solutions. Application layer companies seek to establish their own finetuned and even foundational models, all in an attempt to build up platform moats and capture value.
Ø Use case specific models
Ø Integrations
Application layer
Orchestration
L2 – Finetuned models
L1 – Large models
Ø Proprietary finetuned models &
Hardware layer
Ø Foundational models
….and thus we are left with a game of the few: companies with access to Talent and Capital (Compute).
The structural and sustaining scarcity of compute and talent will peg costs of scaling AI
There is a limited supply of enterprise GPUs: highly concentrated production (60% TSMC market share) & fabs require investments of +$10Bn
Talent
There are only around 10k AI researchers in the field
A100 GPU costs up to $15k >20k GPUs needed to train GPT3
OpenAI: $550M OPEX (2022) with ~500 employees
Without increasing value and ACVs, AI-enabled companies will likely see lower margins vs "pure play" SaaS. Access to capital becomes a competitive moat, as well as actually solving hard problem sets where software solutions can be monetized.
COGS Compute, Storage, Payment Gross Margin
COGS Compute, Storage, Payment
COGS (ML) Training, Inferencing
Gross Margin
With the release of Web GPU for major browsers, even some large models can be run "on the edge" (e.g. Stable Diffusion and Meta's LlaMA). As hardware improves and models become more efficient, some of the inferencing and finetuning will be done on device and thus reducing cloud costs.
Producing state-of-the-art AI innovation is a costly endeavour and could lead to a small amount of dominant players. However, open source models have historically commoditized new AI capabilities in surprisingly short periods of time.
Source: Adapted from StateofAI Report 2022; *Llama model was not intentionally made open-source (leak)
The growing landscape of large language models (LLMs) includes multiple open source models
Large models will likely not monopolize, despite the barriers to scale.
Competitive moats in AI-enabled software companies often stem from capital (team & compute), as well as from data. Both leverage better with a large and existing customer base.
Incumbents can now relatively easily retrofit their applications with L1 and L2 models into their backends. Especially where a UI/frontend does not require radical changes, an incumbent player will be capable of capturing value through AI.
If an incumbent will not have to meaningfully change their user interfaces to capture or create value through AI, their competitive advantage will be strengthened. New entrants should focus on radical UI shifts, as well as addressing new user groups.
We can expect a tidal wave of new (and often incremental) software innovation on top of large models. Obvious problems and "easy to build" solutions will create red ocean markets.
We consulted the Heartcore team and eight external contributors (who are distinguished experts in the AI field) to select a groundbreaking research paper published in the last year and provide insight into why they chose it.
Chosen research paper:
Released in Apr 2021
Generative Agents: Interactive Simulacra of Human Behavior
Stanford University - Joon Sung Park, Joseph C. O'Brien, Carrie J. Cai, Meredith Ringel Morris, Percy Liang, Michael S. Bernstein
Why it’s important:
"In this paper, the team out of Stanford places several generative agents in a shared digital world somewhat similar to the game Sims. These agents, built on LLMs, interact with each other. The interactions are surprisingly realistic, including a coordinated Valentine's day party. If the AI revolution is a continuation of the personal computer revolution, as in a revolution of computation, prediction, and work, then this type of multi-agent interaction is reminiscent of the early days of PC-networking, which eventually led to the Internet."
Chosen research paper:
Released in January 2023
Large Language Models Generate Functional Protein Sequences Across Diverse Families
Profluent, Salesforce - Ali Madani, Ben Krause, Eric Greene, Subu Subramanian, Benjamin Mohr, James Holton, Jose Luis Olmos Jr, Caiming Xiong, et al.
Why it’s important:
"Madani et al. demonstrate how a language model architecture originally designed for code can be adapted to learn the language of proteins. Through large-scale training, they use a protein language model (ProGen) to create artificial protein sequences that encode functionality that is equivalent to or better to naturally occurring proteins. This means we can generate proteins (drugs or otherwise) with desired functions in a far more systematic way than ever before."
Chosen research paper:
Released in October 2022
OpenAI : Bowen Baker, Ilge Akkaya, Peter Zhokhov, Joost Huizinga, Jie Tang, Adrien Ecoffet, Brandon Houghton, Raul Sampedro, Jeff Clune
Why it’s important:
"The research from OpenAI applies semi-supervised imitation learning for computer agents to learn to act by "watching" unlabeled video data. The model was pre-trained with 70k hours of online videos of people playing Minecraft, finetuned with a small amount of labeled data (video labeled with keypresses and mouse movements). The trained model was able to craft diamond tools with human-level performance. Taking this further, complex and sequential tasks could be automated by simply "observing" humans doing the work, e.g., for data entry tasks within or across applications.”
Chosen research paper:
Released in Jan 2023
Why it’s important:
"A research team from Deepmind show that a Reinforcementlearning-based general and scalable algorithm can master a wide range of domains with fixed hyperparameters. By interacting with the game, the model learned to obtain diamonds in the popular video game Minecraft despite sparse rewards, and without human data or domain-specific heuristics. "Learning by doing" across different domains and sparce/delayed rewards is a trait of human intelligence and hence this research presents a potential path towards a "general" AI."
Chosen research paper:
Released in Mar 2023
Alpaca: A Strong, Replicable Instruction-Following Model
Stanford University, Meta- Rohan Taori, Ishaan Gulrajani, Tianyi Zhang, Yann Dubois, Xuechen Li, Carlos Guestrin, Percy Liang, Tatsunori B. Hashimoto
Why it’s important:
"Generative AI models are pivotal for productivity, especially when users can run them on their own hardware. Alpaca showed that a combination of an open-source foundational model and extracted instruction-output pairs can achieve similar performance to text-davinci-003. More importantly, this leap to democratization happened very cost-efficiently (<600 USD). The paper initiated a discussion on how defensible even humanlabeled training data is. It foreshadowed a missing moat of big tech and hints at forthcoming possibilities of AI models stealing from each other."
Chosen research paper:
Released in Feb 2023
LLaMA: Open and Efficient Foundation Language Models
Meta- Hugo Touvron, Thibaut Lavril, Gautier Izacard, Xavier Martinet, Marie-Anne Lachaux, Timothée Lacroix, Baptiste Rozière, Naman Goyal, et al.
Why it’s important:
"Meta's release of LLaMA was important for two reasons. First, it showed that training a smaller model for longer could yield really impressive results - the 13B model outperformed GPT-3, which has 175B parameters. Second, an entire ecosystem has bloomed around LLaMA. Alpaca and Vicuna for starters, but also the efforts to open source a LLaMA-equivalent with commercial licenses, running these models on your laptop and your phone, etc. A lot of progress in the large language model space from 2023 is thanks to Meta and its work with LLaMA."
Chosen research paper:
Released in Mar 2023
Deepmind - Karan Singhal, Tao Tu, Juraj Gottweis, Rory Sayres , Ellery Wulczyn, Le Hou, Kevin Clark, Stephen Pfohl, Heather Cole-Lewis, et al.
Why it’s important:
"Singhal et al present a model designed to tackle the grand challenge of medical question answering with performance exceeding SOTA across multiple datasets. Med-PaLM 2 combines improvements in LLMs with medical domain fine-tuning and novel prompting strategies. The model scored up to 86.5% on the MedQA dataset, surpassing the previous SOTA by over 19%. Combined with the recent progress in multimodal AI, which would allow AI models also to see and hear - we can imagine a world where individuals can access personalized, timely, and accurate medical advice conveniently, empowering them to make informed decisions about their health, improving healthcare access and outcomes for humans across the globe.”
Nicole Büttner CEO atChosen research paper:
Released in Apr 2023
Meta - Alexander Kirillov, Eric Mintun, Nikhila Ravi, Hanzi Mao, Chloe Rolland, Laura Gustafson, Tete Xiao, Spencer Whitehead, et al.
Why it’s important:
"This paper is something like the GPT Moment for Computer Vision. The Segment Anything Model (SAM) trains on more than 1b segmentation masks on 11M images. The model's zero shot capabilities solve many of the computer vision tasks without training data through a prompt, which revolutionizes the field."
Chosen research paper:
Released in April 2023
Efficient Evolution of Human Antibodies from General Protein Language Models
Stanford University - Brian L. Hie, Varun R. Shanker, Duo Xu, Theodora U. J. Bruun, Payton A. Weidenbacher, Shaogeng Tang, Wesley Wu, John E. Pak
Why it’s important:
"Large language models can massively accelerate evolution experiments in the lab, including for clinically-relevant applications. This study used six language models, altogether, that were trained on protein sequences in the UniRef database. The model would suggest mutations - without knowing the target antigen - based on which substitutions have a higher evolutionary likelihood across the six models. The evolved antibodies had improved affinities comparable to those achieved by a state-of-the-art lab evolutionary system (which takes weeks to perform) suggesting that LLMs can massively accelerate clinical development times in some scenarios.”
Darian Shirazi General PartnerChosen research paper:
Released in June 2022
ZeroQuant: Efficient and Affordable Post-Training
Quantization for Large-Scale Transformers
Why it’s important:
"As large language models become even larger, there are significant memory and processing power limitations which increases latency and cost for many applications. This paper introduced a novel technique for post-training Quantization of LLMs by representing parameters in 8 bits rather than 32 bits with minimal accuracy loss and a >5x response time. In time, many products will leverage different optimized models for different use cases and Quantization is one of the major steps towards this reality. While a simple and elegant solution, ZeroQuant has led to a number of other optimization methods such as SmoothQuant and other methods."
Co-founder & CTO at Corti.ai Adj. Professor at DTU
Chosen research paper:
Released in Mar 2023
Are Emergent Abilities of Large Language Models a Mirage?
Ryan Schaeffer, Brando Miranda, Sanmi Koyejo
Why it’s important:
”With the impressive progress and the many use-cases of Large Language Models, it is important to learn what we can expect. As Yann LeCun stated: Auto-Regressive Large Language Models will always hallucinate and it is not fixable. Do they, however, have ‘emergent abilities’: “abilities that are not present in smaller-scale models but are present in large-scale models …”. The authors of this paper presents an intelligent study showing that previous belief that these models possess emergent abilities are wrong, and that it simply has to do with the evaluation metrics. Hence, completing a multiple-choice medical exam is not evidence that a model has emergent abilities.”
Max Niederhofer Partner atChosen research paper:
Released in June 2022
Semantic Reconstruction of Continuous Language from Non-invasive Brain Recordings
Jerry Tang, Amanda LeBel, Shailee Jain, Alexander G. Huth et al.
Why it’s important:
“In this paper, researchers developed a non-invasive decoder that can reconstruct continuous natural language from brain recordings. This allows for the interpretation of perceived speech, imagined speech, and even silent videos. Although cooperation from subjects is still needed, the paper makes us wonder how long this will prevail. Advanced techniques could have the potential to infringe on mental privacy. While fMRI is currently a key tool in this research, the rapid pace of technological advancement means that other methods may eventually supplant it.”
Midjourney
Image Generation US Image Generation UK $89M funding
Game asset generation US $6M funding
Ai powered Design tool DK $19M funding
Product image photo studio tool FR $19M funding
2D to 3D recorder US $28M funding
Audio/ Video editor US $100M funding
Content creation US $131M funding
Synthetic voice API UK >$15M funding
Mobile first retouching tool IL $335M funding
AI first Video editor/ effects suite US $196M funding
Synthetic video avatars UK $67M funding
VFX software for studios US $17M funding
Interactive avatars IL $48M funding
Audio/ Video recording suite IL $47M funding
Generative Music platform US $20M funding
Physics-based animations for games UK >$2M funding $33M funding
AI-powered accounting software US $115M funding
AI-powered contract intelligence US $156M funding
Legal research tool US $65M funding US $26M funding
AI-powered accounting software FR $61M funding
Contract drafting tool BE $11M funding
AI-powered accounting automation US $33M funding
Billing for enterprise law firms US $56M funding
Loan portfolio optimization US $41M funding
ESG insights for investors US $80M funding
Robotics platform US
$222M funding
Robotic logistic automation US $196M funding
Frontline worker protection US $30M funding
Humanoid robots NO $37M funding
Autonomous yard truck management US $58M funding
3PL autonomous fulfilment US $115M funding
Fleet inspection US $196M funding
Autonomous excavators US $112M funding
General purpose robots DE $270M funding
General purpose low code robot arms DE $18M funding
Automated textile inspection PT $27M funding
Frontline worker protection US $22M funding
3PL autonomous fulfilment US $102M funding
Autonomous agriculture vehicles US $58M funding
Robot software DE $123M funding
Robot assistants DE $86M funding
Robot software PL $31M funding